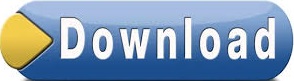

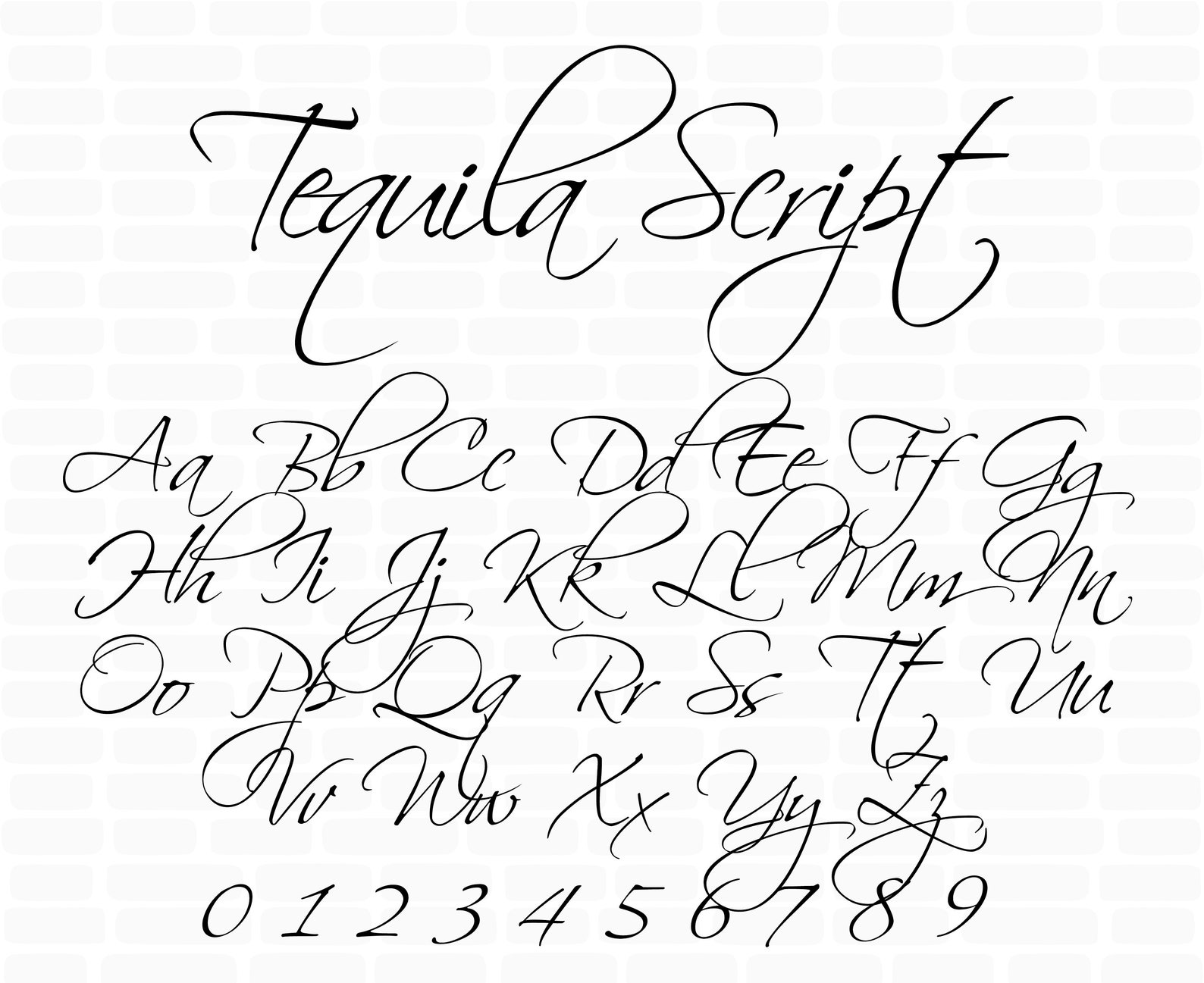
Image captioning using a ConvNet + LSTM model. Since all the parameters of this window are differentiable, the model learns to shift the window from character to character as it writes them Think of this convolution operation as a soft window through which the handwriting model can look at a small subset of characters, ie. In technical terms, it is a Gaussian convolution over a one-hot ascii encoding. Imagine that we want our model to write ‘You know nothing Jon Snow.’ In order to get the information about which characters make up this sentence, the model uses a differentiable attention mechanism. For example, the MDN will choose Gaussian with diffuse shapes at the beginning of strokes and Gaussians with peaky shapes in the middle of strokes.
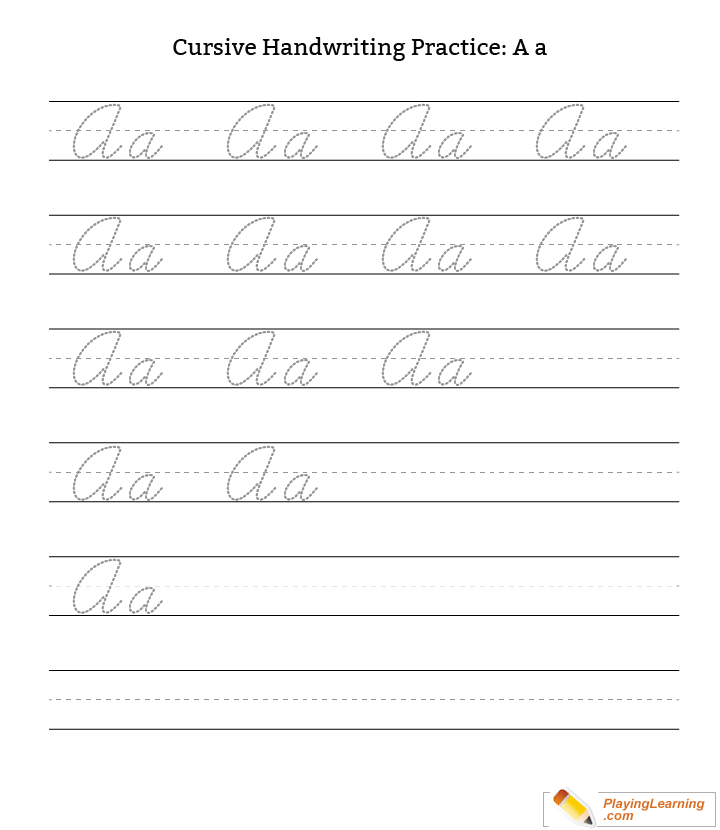
In the handwriting model, the MDN learns to how messy or unpredictable to make different parts of handwriting. Since MDNs parameterize probability distributions, they are a great way to capture randomness in the data. The importance of π: what is the probability the red point was drawn from each of the three distributions? Last year, I wrote an Jupyter notebook about MDNs. Think of \(\pi\) as the probability that the output value was drawn from that particular component’s distribution. They also estimate a parameter \(\pi\) for each of these distributions. Their output parameters are \(\mu\), \(\sigma\), and \(\rho\) for several multivariate Gaussian components. Think of Mixture Density Networks as neural networks which can measure their own uncertainty.
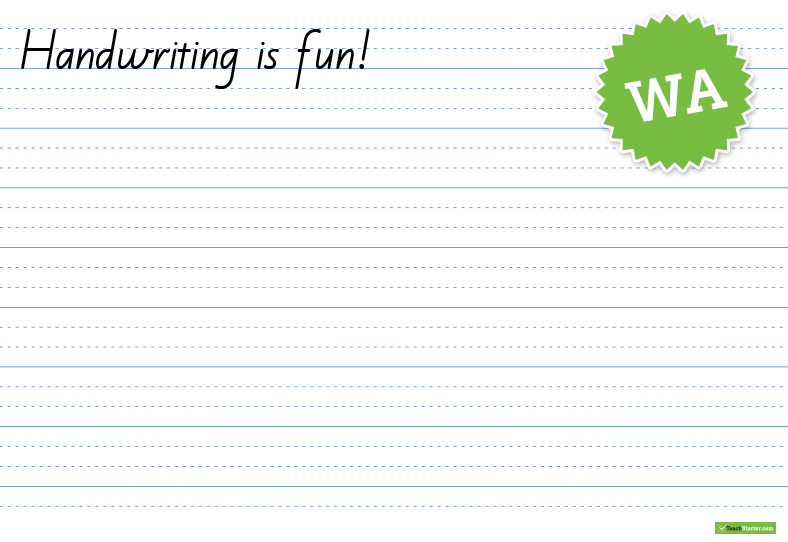
For the purposes of this post, just remember that RNNs are extremely good at modeling sequential data. LSTMs, for example, use three different tensors to perform ‘erase’, ‘write’, and ‘read’ operations on a ‘memory’ tensor: the \(f\), \(i\), \(o\), and \(C\) tensors respectively ( more on this). These networks use a differentiable form of memory to keep track of time-dependent patterns in data. Arrows represent how data flows through the model (gradients flow backwards) The recurrent structure allows the model to feed information forward from past iterations.
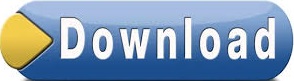